Data Analyst vs Data Scientist: Which Role Fits You Best?
Data Analyst vs Data Scientist: Which Role Fits You Best?
Blog Article
Data Analyst vs Data Scientist: Which Role Fits You Best?
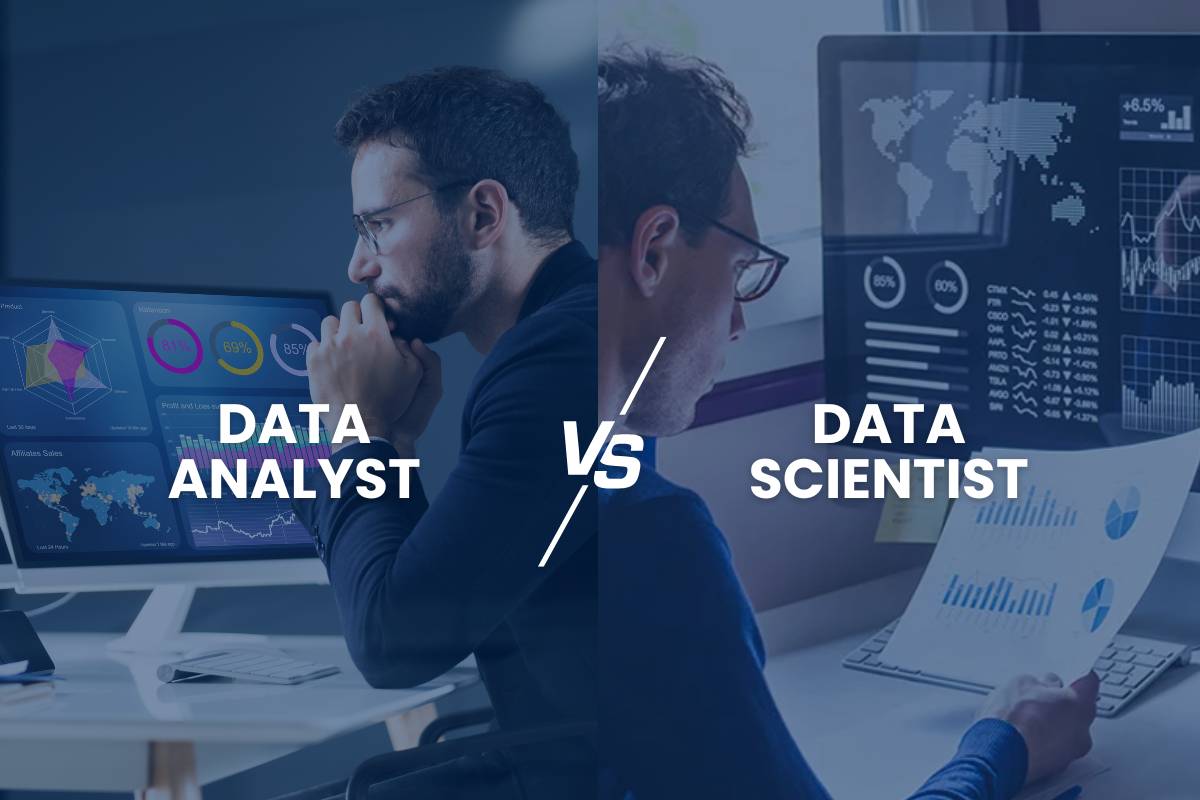
- Source: ashboard. Analyst View more by AndreyPopov from Getty Images
Data is everywhere. The exponential use of the rising technology has led to an increase in data volume. Due to the reliance on data, major businesses are looking for the right talent pool. You can harness this power to predict and solve complex problems with the right skillset and tools. Given this interest, you might be wondering about possible career options. If you are into data exploration, the road leads to two possible outcomes: a data analyst or a data scientist.
These two seem like interchangeable terms, but they are notably different. Organizing their infrastructure has become crucial as businesses evolve at an enormous scale. Thus, investing in the right talent pool. Now, let us look at data analyst vs data scientist, their similarities, qualifications, and the required skillset.
➣ What Is The Role of A Data Analyst?
Data analytics involves analyzing datasets to uncover valuable insights and answer specific questions. When a business needs to understand data, whether gathered internally or from outside sources, it often relies on data analysts.
A data analyst organizes and cleans the data, then looks for patterns and insights to support business leaders in making strategic decisions. Analysts often explore data from the past and present to help companies understand their performance in the market and plan for the future. They excessively use spreadsheets, databases, and visualization tools to organize the raw data.
➣ What Is The Role of A Data Scientist?
Data science is a field that blends many disciplines, such as mathematics, computer science, statistics, and software engineering. It centers on collecting, organizing, and analyzing structured and unstructured data for various business applications.
A data scientist focuses on the data pool. Primary work is designing algorithms and predictive models that data analysts rely on to gather, sort, and interpret data. For example, data scientists are behind designing systems that recommend products, detect fraud, or predict customer traffic. They create tools to extract insights, develop automation systems to reduce repetitive tasks, and build customized data frameworks for their organization.
➣ Data Analyst vs Data Scientist: Understanding The Key Differences
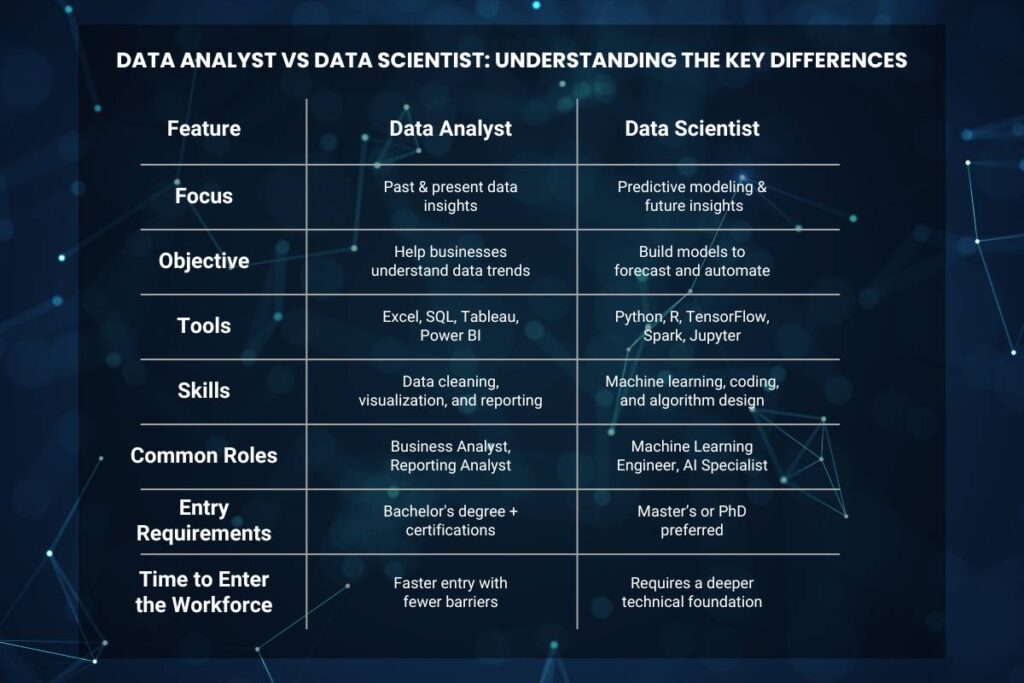
Feature | Data Analyst | Data Scientist |
Focus | Past & present data insights | Predictive modeling & future insights |
Objective | Help businesses understand data trends | Build models to forecast and automate |
Tools | Excel, SQL, Tableau, Power BI | Python, R, TensorFlow, Spark, Jupyter |
Skills | Data cleaning, visualization, and reporting | Machine learning, coding, and algorithm design |
Common Roles | Business Analyst, Reporting Analyst | Machine Learning Engineer, AI Specialist |
Entry Requirements | Bachelor’s degree + certifications | Master’s or PhD preferred |
Time to Enter the Workforce | Faster entry with fewer barriers | Requires a deeper technical foundation |
➣ Data Analyst vs Data Scientist: Project Lifecycle
While data analysts and data scientists aim to make sense of data, how they move through a project is different. Below are the five primary stages in the project life cycle for each role, showing how they approach data-related tasks from start to finish.
• Data Analyst Project Life Cycle
1. Understanding the Business Need: The analyst begins by meeting with stakeholders to understand what questions must be answered. Whether improving customer retention or tracking sales performance, the goal is to clarify the purpose behind the data request.
2. Collecting and Accessing Data: Once the problem is defined, the analyst pulls relevant data from internal databases, Excel files, or cloud systems. This stage may involve SQL queries or working with pre-built dashboards.
3. Cleaning and Preparing the Data: The data must be cleaned before analysis begins. Analysts remove duplicates, handle missing values, and organize the data into a usable format. This process ensures accuracy and reliability in the results.
4. Analyzing and Visualizing Data: Using tools like Excel, Tableau, or Power BI, analysts explore the data to uncover patterns, trends, and correlations. The focus is on providing clear, visual insights that non-technical audiences can easily understand.
5. Presenting Results and Recommendations: The final step involves presenting insights through dashboards or reports. Analysts may suggest actions based on their findings, helping teams make informed decisions supported by real data.
• Data Scientist Project Life Cycle
1. Defining the Problem and Hypothesis: Data scientists start by translating complex business challenges into data problems. They define hypotheses, scope the project, and determine what success looks like—often with a focus on prediction or automation.
2. Gathering and Engineering Data: This phase involves collecting massive datasets from structured and unstructured sources, including APIs, sensors, or log files. Scientists then clean and engineer features that can improve model performance.
3. Building and Training Models: Using machine learning libraries like Scikit-learn, PyTorch, or TensorFlow, they train algorithms on the prepared data. This step includes selecting models, tuning parameters, and validating outcomes through testing.
4. Deploying Models into Production: Once the model performs well, it’s deployed into live systems or applications. This allows the model to make real-time predictions or decisions, such as recommending products or detecting fraud.
5. Monitoring and Improving Performance: After deployment, data scientists monitor the model for accuracy, bias, or drift over time. They refine the model based on new data or changing conditions to maintain its effectiveness.
➣ Data Analyst vs Data Scientist: Qualifications, Skills, and Tools
Education should be the core of pursuing either of these careers. But there will be a slight difference in the path.
A data analyst typically enters the field with a bachelor’s degree in mathematics, statistics, computer science, or economics. Supplementing that with certifications in SQL, data visualization, or Excel can make candidates stand out. Analysts must have strong critical thinking, communication, and business understanding since they often explain data findings to non-technical teams.
A data scientist, however, usually needs a more advanced academic background, often a master’s or even a PhD. Their work involves building models and testing predictions, so knowledge in calculus, linear algebra, and computer programming is key. Learning platforms like Python, R, and TensorFlow are core to their toolkit. They also need experience with handling large datasets and working in cloud environments.
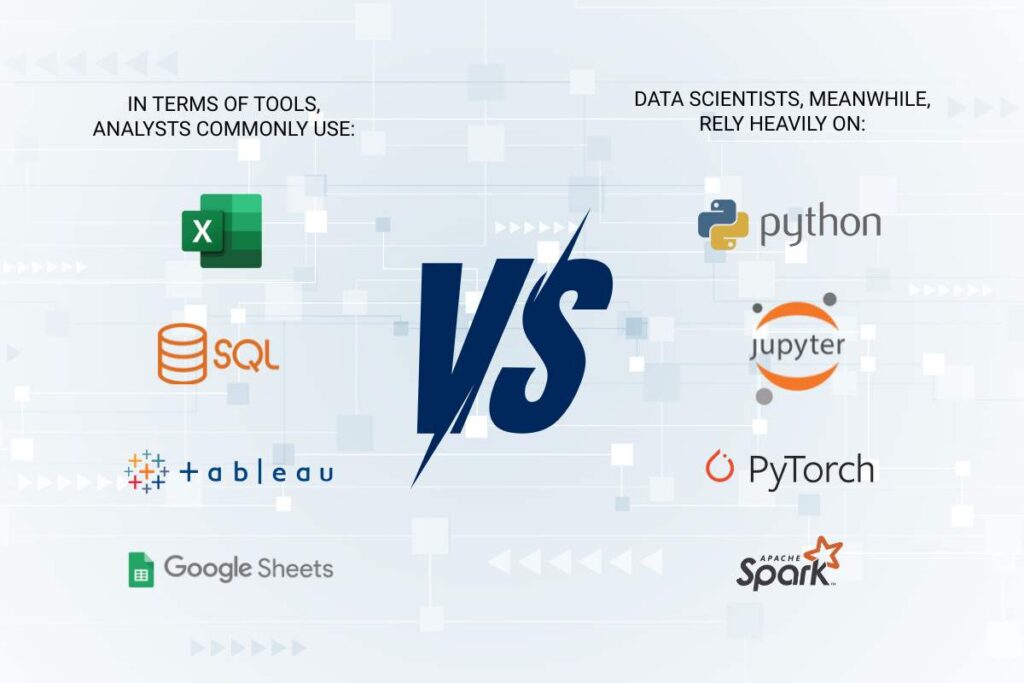
➣ In terms of tools, analysts commonly use:
→ Excel
→ SQL
→ Tableau or Power BI
→ Google Sheets
➣ Data scientists, meanwhile, rely heavily on:
→ Python or R
→ Jupyter Notebooks
→ Scikit-learn, Keras, or PyTorch
→ Big data platforms like Apache Spark
➣ Data Analyst vs Data Scientist: Similarities Between the Two Roles
Despite their distinct responsibilities, data analysts and data scientists share some key similarities. Both roles require a solid understanding of data handling and the ability to draw meaningful conclusions. Both work with data pipelines and rely on structured or unstructured datasets to inform decisions.
Another common ground is collaboration. Analysts and scientists regularly work with business leaders and product teams. They translate technical findings into actionable plans, providing strategic guidance. They also both require strong communication skills, because data has no value at the end of the day unless others can understand and act on it.
Furthermore, many professionals begin as data analysts and gradually shift into data science as they gain more technical skills and experience.
➣ Data Analyst vs Data Scientist: Salary Outlook and Career Opportunities
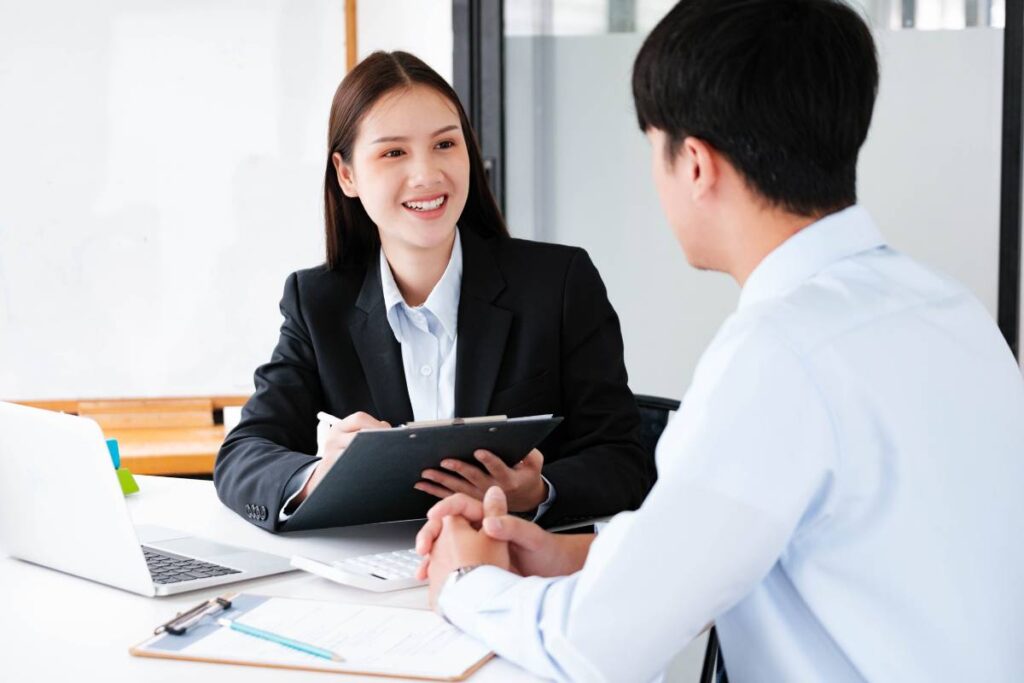
According to recent market insights:
- U.S. data analysts typically earn between $60,000 and $90,000 annually, depending on experience and location. With growth, they may move into senior analyst roles, business intelligence positions, or transition into data science over time.
- Due to their advanced skillset, data scientists earn higher salaries—usually between $100,000 and $140,000, with senior or specialized roles surpassing that. They can progress into Machine Learning Engineer, AI Researcher, or Chief Data Officer roles.
➣ Data Analyst vs Data Scientist: What’s The Final Take?
Concluding the discussion on data analyst vs data scientist, where do you see yourself inclined on the most? Data analytics would be the right choice if you are more interested in raw data to understand business insights. Data science would be the best fit if you are more tech-savvy and enjoy coding and solving complex problems.
Both careers have an outstanding job outlook, allowing you to grow exponentially. Since businesses rely heavily on information, both these roles are perfect to make a significant impact.